Are you a 'data coach' or a 'dogma coach'?
Alan Couzens, MS (Sports Science)
April 4th, 2016
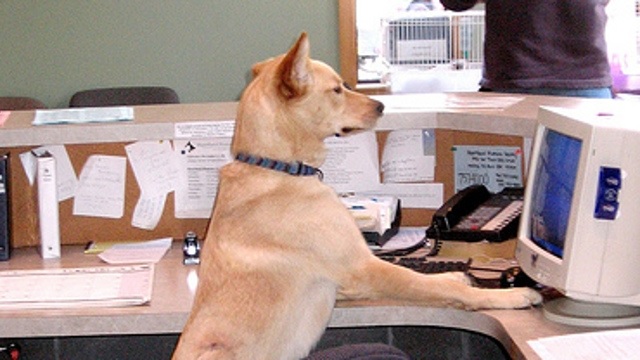
It’s been a while since I’ve had a good grumble, so bear with me... :-)
As technology in sport (& the data associated with said technology) develops exponentially, the pushback associated with the discomfort of change is being felt around the social media sphere. The cries of "Well, we never had the gadgets before and we did all right…” are becoming louder by the day and adopting an almost vehement tone. Why is this?
Math is hard work!
When folks envision becoming a coach, spreadsheets aren’t the first thing that come to mind. No, the picture is more of a coach kneeling side by side with an athlete explaining the training ahead for the day, outlining the details of a plan that has them leaving the track, pool, or field, a better athlete than what they were when they stepped on it. No doubt, this is a huge part of the coaching game but it leaves out one equally important question – what’s behind this plan that the coach is charismatically communicating to the athlete? Put more bluntly, is the plan any good?
Is it based on objective data or subjective dogma?
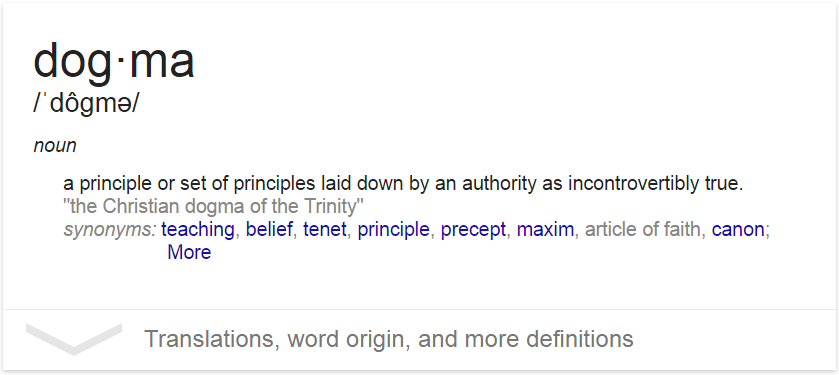
Athletic coaching is still very much a traditionalist profession. Principles and practices have been handed down through coaching generations without a lot of objective assessment of their accuracy or validity to this point. Yeah, yeah, I hear you now – “But my program results in winning athletes, isn’t that enough?” How many winning athletes? What’s your % of winning v ‘losing’ (or injured, dropped out, burned out) athletes? The fact is, when we’re assessing ‘successful’ coaches there is a huge bias in sport to overlook multiple failures for one big win. I'm sure you can think of more than one or 2 'elite' programs/coaches that match this description.
And so we’re left with these dogma based ‘set in stone’ programs, ‘validated’ only by the 2% success stories, that athletes are perpetually churned through. Some (the genetically fortunate) make it & some don’t. “Oh well, that’s the cold, hard reality of competitive sport.”, you hear. In my mind, that’s the lazy answer. In my mind, in the modern era, there is another, a smarter, a better, way…
My newest passion is machine learning (or artificial intelligence if you want to get a little more ‘sci fi’ about it :-) I’m currently reading a great book on the subject entitled “The Master Algorithm” by Pedro Domingos. The following quote from said book expresses this difference in old v new approaches most eloquently…
“Every algorithm has an input and an output: The data goes into the computer, the algorithm does what it will with it & out comes the result. Machine learning does the opposite of this, in goes the data & the desired result & out comes the algorithm that turns one into the other.”
That’s worth a re-read…
Traditional – program is largely set. Inputs and outputs are variable
Modern approach – program is variable & builds around the inputs in order to maximize the chances of a successful output.
Old school (dogma based) everything – coaching, finance, education, medicine is about running individuals through a program. 'New school' is about defining a result and building the program (/portfolio/syllabus/treatment plan) to get to the target result for that individual.
In the domain of coaching, this means collecting both quantitative & qualitative data, from the individual, to objectively answer things like…
- If athlete x does y amount of training load, will z = increased performance or will z = sick & injured? And, taking it a step further, what’s the probability of each outcome?
- Just as importantly for AG athletes – how much extra performance bang will I get if I increase training load by x %? In a time investment/scheduling sense, is this realistic & is it worth it?
- If I am going to increase training load, will I get the most response (& mitigate injury/illness/burnout risk) by increasing volume or intensity for this athlete?
And the grand-daddy of ‘em all…
* What combination of all of the above factors, volume, intensity, training zones, timing of the load will lead to the highest performance on race day? i.e. what is the optimized training model for this individual?
Make no mistake about it, coming up with good, personalized, answers to the above questions for each individual athlete is no longer science fiction. With almost every athlete perpetually toting the ‘super-computer’ that is a 920XT or equivalent, we have a huge mass of data, these days, to do a really good job of answering these questions. I talk more about the specific 'data science' behind how we can go about using the data at our disposal to answer the above questions in part 2, here But, in the ‘real world’ of coaching, 3 things are slowing this advancement. We need…
- First and foremost, a dramatic paradigm shift away from valuing the 'old school' shotgun approach of let’s throw a bunch of athletes into a program and whoever makes it great, whoever doesn’t ‘oh well, they didn’t have it’
- A serious ability to organize all of the incoming data. This is the current challenge. The folks who are receiving the data (the coaches) are overwhelmed and don’t know what to do with it.
- Some math chops! To make meaningful sense of the relationships between the data, it’s time to dust off some of the old math texts – algebra, probability, series & sequences, statistics etc.
If it sounds like a lot of work, you’re right. It’s much easier to join the ‘old school’ cries of “well we never did it like that in my day” but in every competitive field, 'old school' is going the way of the Dodo. Everything is becoming individualized and optimized at a break-neck rate – from your spam filter, to the marketing that you receive, to the books you read, to your financial portfolio, to the words that you use in your texts & emails. And now, your training plans! In all fields, technology is getting better, individualization and optimization systems are getting better, people are getting smarter. Or, again in the words of Mr Domingos...
“A frequently heard objection (to the introduction of data science) is that data can’t replace human intuition. In fact, it’s the other way around: Human intuition can’t replace data! Intuition is what you use when you don’t know the facts and since we often don’t, intuition is precious. But when the evidence is right before you, why would you deny it?”
In any field, with the amount of data now available & accessible, if you want to truly stay competitive, you’re going to have to keep up. Attachment to dogma & grumbling about 'the good old days' just isn’t going to cut it…
Time to adapt. Time to evolve. Time to change. Time to learn new things.
Train smart,
AC
Tweet