Calculating your coaching "suck factor"
Alan Couzens, M.Sc. (Sports Science)
Updated April 13th, 2017
As Happy Gilmore showed us, it’s not fun being told we suck! But sometimes we need to objectively realize just how much we suck in order to get better. Take coaching for instance....
In my mind, coaching is largely about your ability to forecast…
Think about this for a sec. An athlete comes to us with a goal, a destination in mind and their big question to you will be, “OK Coach, so what do I need to do to get there?” Your ability to answer that comes down to what you've observed with similar athletes on similar paths before. A lot comes down to your ability to size up this athlete & given a particular training plan, your ability to accurately forecast his/her performance in relation to their goal. If your 'guesses' as to where the athlete will be after following your plan for a period of time aren't very good, your chances of continuing to work with that athlete, & at a larger level, as a Coach, aren't very good. So, we might say, a lot of your overall efficacy as a coach comes down to your answer to this one question…
Are you a good forecaster?
I’m reading a book at the moment about “Superforecasting: The art & science of prediction” & it has been nothing short of eye opening. Not only in elucidating some of the unique skills that these people who have the ability to predict future events well beyond the population average have, but also, probably more so, in our general desire as a species to not want to know just how bad we are at prediction!
We like certainty. We also like control. In our occupations/vocations we like to believe that we have a very large impact on the result. In order to protect this bias, we often don’t put a lot of effort into quantifying the actual impact we have. This is an ironic problem because, as we tell our athletes, the first step in improving is identifying where we currently are.
The current situation, in the words of the author, Phillip Tetlock…
“In so many high stakes endeavors, forecasters are groping in the dark. They have no idea how good their forecasts are in the short, medium, long term – and no idea how good their forecasts could become. At best, they have vague hunches. That’s because the forecast-measure-revise procedure operates only within the rarefied confines of high-tech forecasting, such as the work of macroeconomists or marketing and financial professionals..More often than not, forecasts are made & then…nothing”
How well does the above description apply to the current situation in coaching?
Coaches are reluctant to quantitatively define fitness because it conveniently leaves room for interpretation. If fitness had the same absolute currency as money, the efficacy of any strategy would become readily apparent and the truth would surface as in the above example of finance. If you see your bank account continually going down, no amount of charismatic “well, the conditions didn’t suit you today, we’ll get ‘em next time champ” is going to prevent you from looking for another financial adviser. This fact means that, in the world of high end finance, the ones who are actually good at forecasting the link between strategy and positive, quantifiable returns flourish, while the 'good talkers’ die out.
A similar scenario has occurred in medicine, though it took a while: It was not until the 20th century that a rational approach to saving lives predominated. Until very recently, the travelling medicine man shows – using their charisma and sales tactics to falsely bolster the efficacy of their ‘health tonics’ abounded. In fact, the book outlines a 1921 (less than a century ago) newspaper article with the following quote from a physician that hints at the potential change afoot from medicine as an 'art' to medicine as a science…
“Is the application of the numerical method to the subject matter of medicine a trivial and time-wasting ingenuity as some hold or is it an important stage in the development of our art, as others proclaim it?”
Obviously, we know now that the answer was the latter. Fortunately (for us all) the quacks who ignore science in favor of sticking with their home potions, have by and large fallen by the wayside and been replaced by numerically validated treatment strategies. Point being, though, it was not until serious evaluation via math and numbers entered the picture that true progress was made & that the transition from medicine being described by its own practitioners as an ‘art’ actually moved to its confirmed current status as a science. It was not until statistical analysis was applied to carefully controlled and randomized trials that we started to get to the truth of what actually works & due to the stakes involved, what 'worked' became the prevailing strategy.
The above bears a striking resemblance to current attitudes in the press with regards to machine learning & artificial intelligence in the current era, doesn’t it? Perhaps (hopefully) the ease in application of those technologies to analyze the efficacy of various strategies in a faster, easier & more practically applicable way to a much broader array of fields will have a similar revolutionary effect in cutting through the sales and marketing B.S. to uncover the truth. But it will be painful because it brings the inadequacies of our current approaches to the forefront (in a similar way to the medicine men of the turn of the century) & it brings in a tough word for coaches and athletes to deal with – doubt.
In the words of mathematician George Box…
“All models are wrong but some are useful.”
Yes, when we move to the realm of mathematical models, we move from the comfort of perceived certainty to the anxiousness of percentage chance. Certainty gives warm and fuzzy’s, chance & the doubt that it brings makes us a bit on edge. It is no coincidence then that many coaches are (false) brokers in certainty! Guru-like certainty, even if it is a lie, has its emotional appeal. Of course, the 'perceived' word in the above is key. Just because an athlete or coach may feel certain about a particular course of action leading to a guranteed result, it doesn't make it so! If we look at the results that spring from a given course of action, it’s never 100%. And the reason for that is simple – our actions are based on (often incomplete or poorly defined) mental models that we have of previous occurrences. In the words of George - ALL models are wrong! They’re only references. We don’t have a 100% model of what will happen in the current occurrence because, in the words of Heraclitus…
“No man ever steps in the same river twice, for it’s not the same river and he’s not the same man”.
Every new athlete & every new season is a completely new experience - a completely new river to navigate. However, as George points out that doesn’t mean that references to previous similar experiences (/rivers) in the form of models (maps) won’t be useful. In terms of getting us closer to the target in predicting future outcomes, knowing some typical signs of rapids or an upcoming waterfall can be very useful! The key then, is to have the humility to realize that our models will never perfectly describe the current reality. We will never (can never) know all of the variables that go into a result but this shouldn't make us turn away from them as 'worthless', nor should it stop us from leveraging the power that, even an incomplete, imperfect, model brings to the table.
So, this brings us to the key question of this post:
Just how accurate & useful are your models?
How ‘right’ are your assumed links between types and volumes of training and results? What percentage of time do things pan out as forecasted within a reasonable error range? Do you even know?
“Well, sure I know. Billy was state champion last year. My program works” Well, considering the number of factors involved in athletic success, it's a possibility that Billy might have done well in spite of your program. Just for comparison, how did Joe-Bob do? What about Curly Sue? What about Moe with the gimpy leg? I notice you don’t talk about them quite as much :-) In this day and age, the “this one time at bandcamp..” anecdotes aren’t going to cut it. We want numbers. Because, in the words of Dr. Tetlock…
“even a dart throwing chimp hits the bulls-eye every once in a while.”

If we really want to improve this coaching game, we need to start to statistically tease out the true efficacy of our approaches – the results that are due to true difference in protocol vs those that are purely the result of “dart-throwing chimp” chance mixed with good marketing. If we really want to get better, we need a ready metric that you can apply across your athletes that tells you how close what you say is going to happen and what does actually happen are!
Fortunately, we live in the golden age of machine learning and there is a lot of research going on into how we can quickly and efficiently build better predictive models. At the initial, most basic level, this begins with identifying a ‘cost function’ or measure of the performance of your model – how far away from reality your model actually is.
When dealing with regression problems, i.e. forecasting continuous variables (like race times for instance :-) on the basis of one or more inputs, a good performance measure is the RMSE (root mean square error). This tells you the standard deviation of the errors that the system makes in its predictions. In other words, it measures how far your predictions fall from real life, i.e. your suck factor.
For instance, if my RMSE was 5% it would mean that approximately 2/3 of the predictions I make fall within 5% of the real world number (/race time, test result etc).
This 'performance forecasting suck factor' (RMSE) that calculates just how wrong your predictions are is calculated as follows…
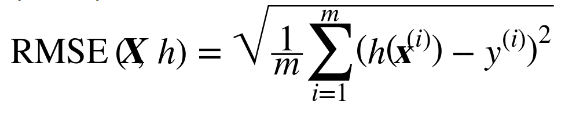
Where h(x) (also known as y-hat) is your (model) hypothesized value for y for a given x, y is the actual observed value & m is the number of observations
So, if you have a column of what you expected performance to be & you compare with the athlete's actual performance on that date, it’s very simple to calculate the RMSE & identify how good (or bad) your model and your predictive abilities are! On a more positive note it is then equally simple to tweak your model in an attempt to lower this RMSE, learning a few things in the process about the actual link between different training interventions and performance outcomes, & coming up with a model that better predicts future performances.
At the simplest level, if after comparing your predictions with what actually occurred you notice that, say, globally your predictions are about 10% too optimistic, you can adjust your 'optimism constant' so that you take 10% away from all your predictions before making them (& become a bit more of a grumpy old man like me in the process :-)
A more detailed application of the power of this predicted-v-actual comparison: The following 2 columns show predicted fitness vs measured fitness (in the form of VO2 scores) for an athlete that I work with & the RMSE at the bottom.
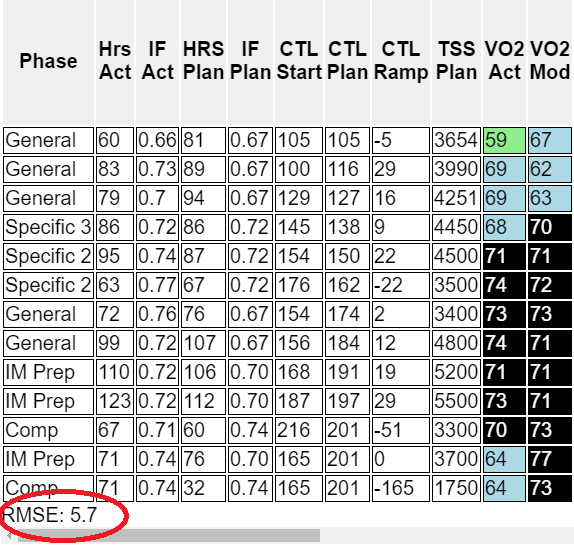
In this case, the RMSE is 5.7, meaning ~2/3 of the samples fell within 5.7 of the model. You can see that the typical numbers for this athlete are in the 70's, so this represents an error in the predictive model of 7-8%. You can also see that the model failed after Kona in the second peak of the year when it failed to account for post Ironman fatigue and significantly overstated the expected VO2 of the athlete (this is a common issue with the Bannister model which doesn't differentiate between acute and chronic forms of fatigue). Apart from this time, though, it lines up quite well.
So what's a good number?
This bring us to another important distinction between practical vs statistical significance. In this day and age, statistical significance is pretty darn easy to come by and the reason is simple – it typically rewards larger samples and in this age of big data, it’s easy to come by some VERY large samples! So let’s move on from statistical significance to practical significance.
Is an RMSE of 5% practically significant? If I were able to say to you – if you follow this program, there is a 67% chance that your race time will fall between 9;30 and 10:00 is that practically significant? Probably. Maybe if we bring it back to 9:00-9:30 it might even be more practically significant to the goals of a lot of athletes I know ;-)
Don’t get me wrong, in the eyes of the 2 out of 10 athletes who fall on the wrong side of the model, you're still gonna suck - you Jackass! :-). But at least you now are able to quantify just how much you suck and quantifying how much you suck is the first step to tweaking things so you can suck a little less on the next iteration.
So, why don’t we play with the model a little bit to see if we can improve its predictive power...
Making your model better (sucking a little less)..
Based on my experience in playing around with this, there are 3 particular ‘tweaks’ to the model tend to yield big results in terms of the accuracy of predictions for the individual athlete…
1. Individualize – The first and most important is to individualize the parameters of your model to the individual athlete. In other words, by identifying your athlete as a ‘natural’, ‘realist’ or ‘workhorse’ & adjusting the coefficients accordingly, you can greatly increase your ability to predict a given performance based on the load. As a group model, my RMSE is in the range of 10-15%. If I bring that down to an individual model, I can cut that error in half to 5-8%.
2. More features – This is something that I’ve talked about in a previous post. We are still slaves to the Bannister model which essentially deals with one input – load (whether in the form of Bannister’s original TRIMPS or Coggan’s modified TSS) By moving away from this to incorporate additional features – separating load into its volume and intensity components, adding life factors like stress, & sleep we can significantly improve the performance of our model.
3. Different algorithms – We’re not limited to the 2 factor polynomial of the Bannister model any more. It’s 2017! We can simply and quickly implement new, better algorithms like Random Forests, SVMs, Neural Networks or even plain old polynomial regression but with more than just load as an input, to come up with a model with many more facets that can better describe the relationships in the data.
I’ll be honest, playing with & tweaking the 3 items above to incrementally improve your models can be a lot of fun! But the first step is going to be painful – looking at your previous data training v performance data objectively to discover, quantify & admit(!) just how wrong you actually have been. Painful as it is, though, it’s the only honest route to truly improving your ability to tailor training to maximize the individual athlete’s chances of achieving their goals…
In summary, don't suck: Forecast -> Measure -> Revise
Coach smart,
AC
TweetDon't miss a post! Sign up for my mailing list to get notified of all new content....